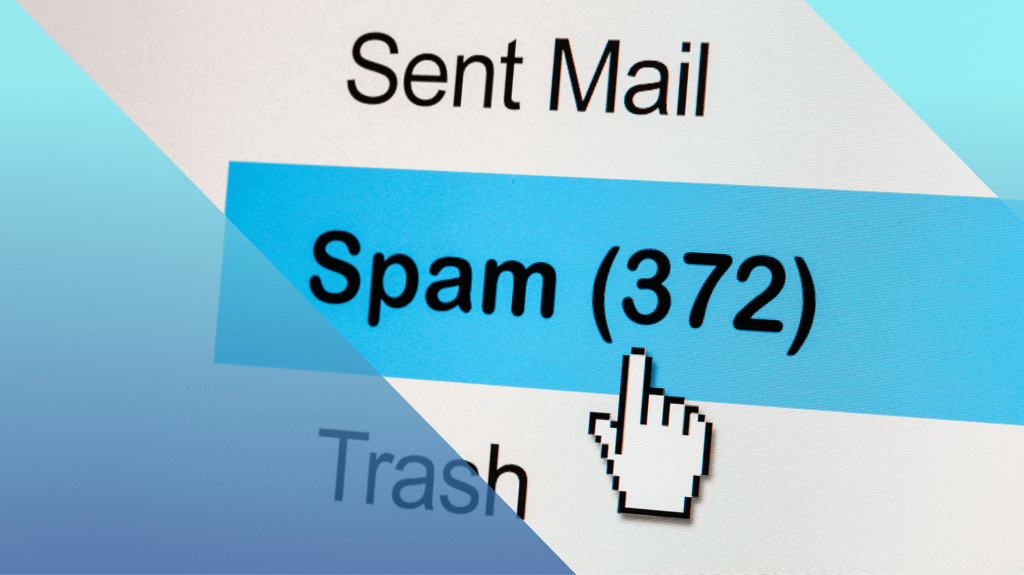
Explained: Bayesian spam filtering
Bayesian spam filtering is based on Bayes rule, a statistical theorem that gives you the probability of an event. In Bayesian filtering it is used to give you the probability that a certain email is spam.
The name
Named after the statistician Rev. Thomas Bayes who provided an equation that basically allows new information to update the outcome of a probability calculation. The rule is also called the Bayes-Price rule after the mathematician Richard Price, as he recognized the importance of the theorem, made some corrections to Bayesâ work and put the rule to use.
Spam
When dealing with spam the theorem is used to calculate a probability whether a certain message is spam based on words in the title and message, learning from messages that were identified as spam and messages that were identified as not being spam (sometimes called ham).
False positives
The objective of the learning ability is to reduce the number of false positives. As annoying it might be to receive a spam message, it is worse to not receive a message from a customer just because he used a word that triggered the filter.
Scoring
Other methods often use simple scoring filters. If a message contains specific words a few points are added to that messagesâ score and when it exceeds a certain score, the message is regarded as spam. Not only is this a very arbitrary method, itâs also a given that this will result in spammers changing their wording. Take for example âViagraâ which is a word that will surely give you a high score. As soon as spammers found that out they switched to variations like âV!agraâ and so on. A cat and mouse game that will keep you busy creating new rules.
Learning
If the filtering is allowed for individual input the precision can be enhanced on a per-user base. Different users may attract specific forms of spam based on their online activities. Or what is spam to one person is a âmust-readâ newsletter to the next. Every time the user confirms or denies that a message is spam, the filtering process can calculate a more refined probability for the next occasion.
Poisoning
A downside of Bayesian filtering in cases of more or less targeted spam is that spammers will start using words or whole pieces of text that will lower the score. During prolonged use, these words might get associated with spam, which is called poisoning.
Bypasses
A few methods to bypass âbad wordâ filtering.
- The use of images to replace words that are known to raise the score

- Deliberate misspelling, as mentioned earlier.
- Using homograph letters, which are characters from other character-sets that look similar to letters in the messagesâ character set. For example the Omicron from the Greek which looks exactly the same as an âOâ, but has a different character encoding.
Conclusion
Bayesian filtering is a method of spam filtering that has a learning ability, although limited. Knowing how spam filters work will make it more clear how some messages get through and how you can make your own mails less prone to get caught in a spam filter.
Links:
Evaluation of Bayesian Spam Filter and SVM Spam Filter
Machine Learning Techniques in Spam Filtering
Pieter Arntz